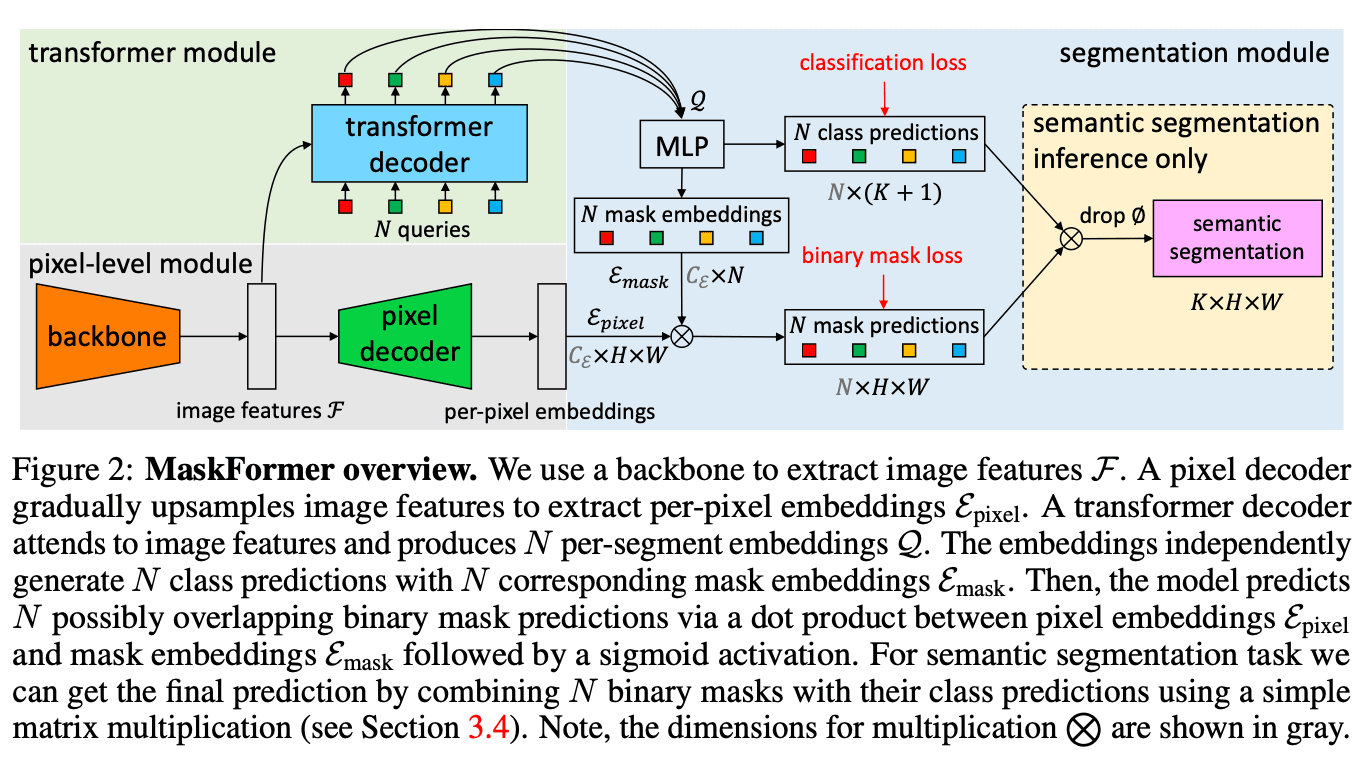
MaskFormer: Per-Pixel Classification is Not All You Need
An in-depth analysis of the revolutionary MaskFormer paper from NeurIPS 2021 that fundamentally changed how computers understand images by shifting from per-pixel classification to masked classification.
Introduction
The MaskFormer paper suggests a potential shake-up of how computer vision understands images. For many years, frameworks for detailed image understanding, specifically semantic segmentation, revolved around pixel-by-pixel classification where each pixel gets classified individually. This paper argues that there is a better and more unified approach.
Semantic segmentation is like looking at a picture and identifying that this whole area is a road, this is a tree, this is the sky-line. It's important because if we have a self-driving car, we not only need to know where a tree, a car, a curb or the road is but we need to know the exact location of it—we need to know precise boundaries of things.
Pixel-by-Pixel Classification
Traditional pixel-by-pixel classification treats each pixel as its own datapoint. The algorithm looks at a picture, its color, its texture, the surrounding pixels for context, and tries to assign a class to it from a predefined list.
A major step forward with this was the development of Fully Convolutional Networks (FCNs), which allowed for the image to be processed in one go and output a classification for each pixel.
However, the MaskFormer paper states that this pixel-by-pixel classification approach has significant limitations.
Limitations of Pixel-by-Pixel Classification
- Difficulty identifying varying numbers of objects within an image
- Challenges in telling apart distinct instances of the same class of objects
These limitations exist because pixel-by-pixel classification labels each pixel in sort of an isolation, assuming a fixed number of categories at each pixel. It can identify that there is a person but can't separate if there are multiple people in the frame.
Instance-Level Segmentation
Instance-level segmentation identifies unique objects and draws a clear boundary around each unique object.
For example, in a scenario with 3 distinct apples in an image:
- Per-Pixel Classification would paint all apple pixels, without distinguishing that they are unique and would not give an outline per unique apple.
- Instance-level segmentation would do everything the per-pixel approach does but also add distinct outlines per apple.
What's interesting, and a key focus of this paper, is that for segmentation another approach has become dominant: masked classification.
Masked Classification
Instead of classifying individual pixels, masked classification classifies entire regions or masks. To each mask, we assign a class label.
This introduces flexibility as the model can identify any number of masks, which is crucial for picking out individual objects in instance segmentation.
Models like Masked R-CNN or DETR have shown success using this masked method not just for instances but even for panoptic segmentation, which cleverly combines both semantic "stuff" (sky, grass) and instance categories (cars, people).
Historical Context
Even before the rise of Fully Convolutional Networks and the dominance of per-pixel classification, some of the most performant segmentation techniques like O2P and SDS were using the masked classification approach.
It's almost as if the field took a detour focusing on pixels and is now refocusing back on classifying regions directly.
As the field focused on per-pixel classification, evaluation metrics like Mean Intersection Over Union (mIoU) naturally aligned with the per-pixel evaluation framework.
This research strongly indicates that mask classification is a more fundamental and versatile paradigm capable of capturing both semantic and instance segmentation within a single framework.
MaskFormer Architecture
Key Innovation
The central idea is to take existing per-pixel classification models and ingeniously transform them into masked classification models.
It's about leveraging the sophisticated capabilities of per-pixel feature extraction but then processing these features to directly predict region-based masks and their associated class labels.
MaskFormer achieves this through a 3-layer architecture:
Modules
- Pixel-Level Module: Extracts rich, detailed feature information (per-pixel embeddings) for every single location in the image. Per-pixel embeddings are highly descriptive signatures for each pixel, including color, texture, spatial relationships, etc. It's flexible, incorporating various backbone architectures like ResNet or Swin Transformer.
- Transformer Module: Uses an encode-decode architecture, similar to what has revolutionized NLP. It takes picture features from the per-pixel embeddings module and processes them in conjunction with learned per-segment embeddings (educated guesses). The Transformer Decoder refines these initial guesses, allowing the model to predict all segments in the image in parallel.
- Segmentation Module: Takes refined per-segment embeddings and generates two crucial outputs:
- Class Prediction: Includes a "No Object" option for segments that don't correspond to any category.
- Mask Embedding: Combined with the per-pixel classification embedding from the first module using a dot product, then passed through a Sigmoid Function to give us the binary mask prediction and the precise shape of the predicted segment.
The process can be summarized as:
- Start with detailed pixel-level understanding
- Use the transformer to propose and refine potential objects and regions
- Generate both a label and a precise boundary for each proposed object
MaskFormer does all of this using the same underlying backbone model, the same way of learning the loss function, and the same training procedure for both broad scene understanding (semantic segmentation) and identifying individual objects (instance-level segmentation).
Traditionally, these tasks had to be approached as distinct problems requiring specialized architectures and training strategies. MaskFormer offers a unified solution, using a combination of Cross Entropy Loss for classification and Binary Mask Loss (a blend of Focal Loss and Dice Loss) to ensure highly accurate masks.
Inference Process
MaskFormer produces a collection of probability-mask pairs. For every pixel in the image, the model finds the predicted pair where both the class probability (confidence about the category) and mask probability (likelihood of the pixel belonging to that mask) are high. Pixels associated with the same high-confidence probability and mask probability are grouped together to form a segment, which gets assigned the predicted class label.
Handling Both Semantic and Instance Segmentation
For semantic segmentation, if the model predicts multiple segments belonging to the same category (e.g., several predictions for "building" in different parts of the image), the inference strategy merges these segments into a single region.
For instance-level segmentation, because each predicted segment originates from a unique probability-mask pair, the model can easily distinguish between individual instances of the same class (e.g., each car in a parking lot would correspond to a different predicted mask).
Semantic Inference Strategy
For semantic segmentation tasks, the researchers discovered that considering all predicted probability-mask pairs for each class at every pixel often leads to better results than making a definitive assignment to a single pair.
For each possible class at each pixel, they sum up the probabilities of all predicted masks belonging to that class, then choose the class with the highest probability. Interestingly, they found that directly maximizing the per-pixel class likelihood degrades performance, suggesting that the mask-based training approach better guides the model's learning.
Performance Results
Large Number of Classes
On the challenging ADE20K dataset with 150 semantic categories, MaskFormer achieved a new benchmark when using a Swin Transformer as its backbone. This surpassed per-pixel classification methods using the same backbone but with more optimal computation and fewer parameters.
Low Number of Classes
While not showing a huge leap in mIoU for datasets with fewer classes, MaskFormer showed improvement in PQST (treating all categories as "stuff" like streets and sidewalks), suggesting better ability to identify well-defined regions.
On datasets like Cityscapes (19 classes), MaskFormer's mIoU was comparable to the best per-pixel classification methods. The researchers note that on such small datasets, it may be difficult to achieve fine-grained pixel accuracy comparable to methods optimized specifically for that.
Panoptic Segmentation
MaskFormer set a new state-of-the-art on the COCO dataset, achieving high Panoptic Quality and outperforming previous top models like DETR, even when using DETR's own post-processing techniques. It also showed strong performance on the ADE20K panoptic dataset.
Why Does It Perform So Well?
The performance gains weren't solely attributed to changes in the loss function. The researchers compared MaskFormer to their modified per-pixel classification model using the same mask-based loss functions, and MaskFormer still showed significant improvements, highlighting the benefit of the mask-based paradigm itself.
Bipartite Matching
The researchers investigated the impact of bipartite matching and found that using this dynamic matching process led to better results compared to simpler, more static matching strategies.
Number of Queries in the Transformer Module
They tested various numbers of initial "educated guesses" in the transformer module and found that 100 queries was a sweet spot for any dataset. This suggests that each query is capable of capturing masks from different categories, not necessarily one query per object.
Surprisingly, even a simplified MaskFormer with a single transformer decoder layer performed well for semantic segmentation, outperforming some existing models. More transformer layers proved more critical for instance-level segmentation tasks like panoptic segmentation, likely because they aid in distinguishing and separating individual object predictions.
Bounding Boxes vs. Masks
One particularly interesting finding was the comparison between matching based on predicted masks directly versus using bounding boxes (as in models like DETR). Matching based on masks directly yielded better performance, especially for "stuff" categories like sky and grass that don't have well-defined rectangular shapes. These regions are large and irregular, making mask-based matching less ambiguous than trying to fit a rectangular bounding box around them.
Computational Efficiency
The researchers also pointed out that calculating mask predictions is more computationally efficient than DETR's box-based approach.
Main Takeaways
MaskFormer convincingly demonstrates that masked classification isn't just a valuable alternative to classical image segmentation but in many ways a superior approach, especially when dealing with a large number of object categories.
It offers a unified framework that can handle both broad scene understanding (semantic segmentation) and identification of individual objects (instance-level segmentation) using the same underlying model, learning process, and training procedure.
This simplification represents a significant step forward, offering a potentially more powerful and efficient way for computers to interpret the visual world.
The paper demonstrates a significant shift in how we approach image understanding and has important implications for AI applications that depend on detailed visual comprehension, such as autonomous vehicles needing to precisely identify every object and region on the road, or robots interacting with complex environments.
Key Concept
Instead of the pixel being the primary unit, masked classification focuses on predicting a set of binary masks (precise digital stencils) for each potential object. The backbone (initial part of the neural network) extracts useful features from raw images, which are then passed to the MaskFormer model.
The most impressive achievement is that the same underlying mechanism can understand both broad scene categories and individual objects within that scene with great accuracy.